Welcome to my slice of the internet. I write a newsletter around the intersection of business, technology, career growth, and leadership.
Don't miss out. Subscribe today to stay up-to-date and receive free weekly updates straight to your inbox.
The Race to the Bottom
The silent and relentless erosion of corporate soul.
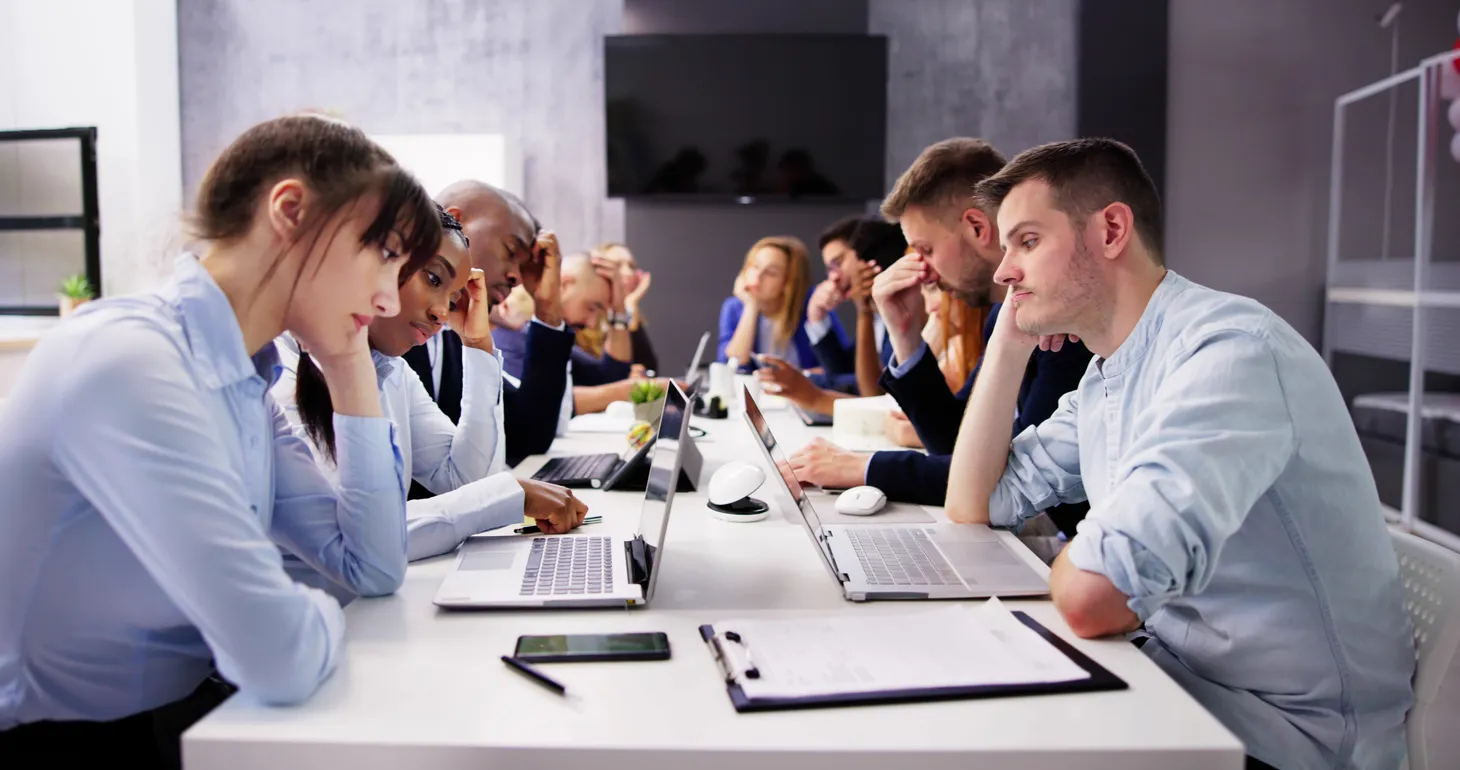
Navigating the Upside Down as a Technology Leader
Ideas for leading technology organizations with confidence and creativity.

Call to Adventure
My career's next chapter.

Artist for a Day
The future of creative output is multiplied by AI.

From Bricks to Castles
What happens when our heroes come up short? Common wisdom around heroes, role models, and mentors is incomplete.

Subscribe for Free
Want to stay ahead of the curve? Subscribe now to receive the latest updates, actionable insights, and thought-provoking ideas around business, technology, and leadership straight to your inbox.